
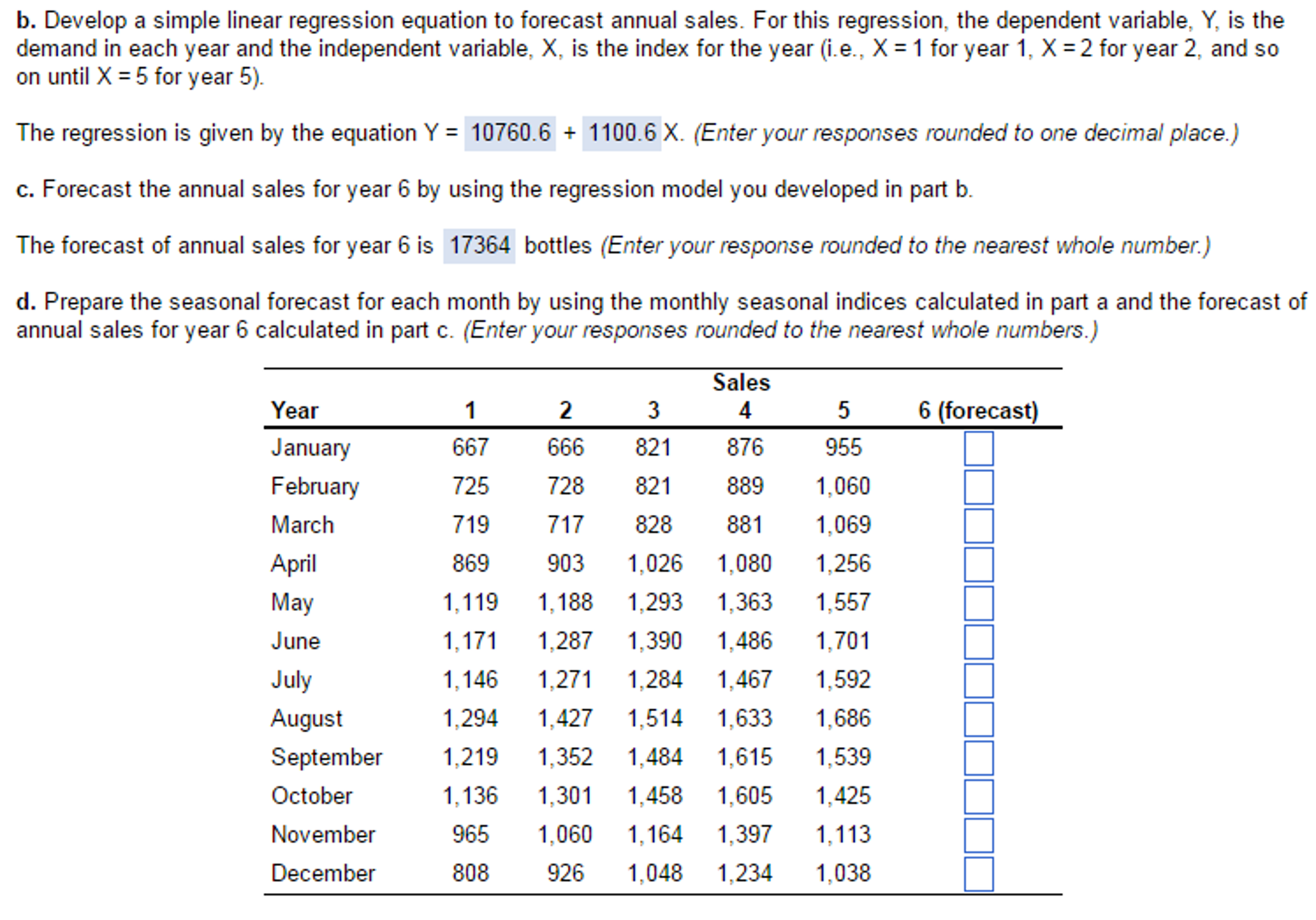
Subsequently, it will further be assumed that ε is distributed normally. Thus, it is assumed that ε is observed as independent and identically distributed random variable with mean zero and constant variance q². For example, the variables may be qualitative, inherent randomness in the observations, and the effect of all the deleted variables in the model also contributes to the differences. Several reasons can be attributed to these differences.
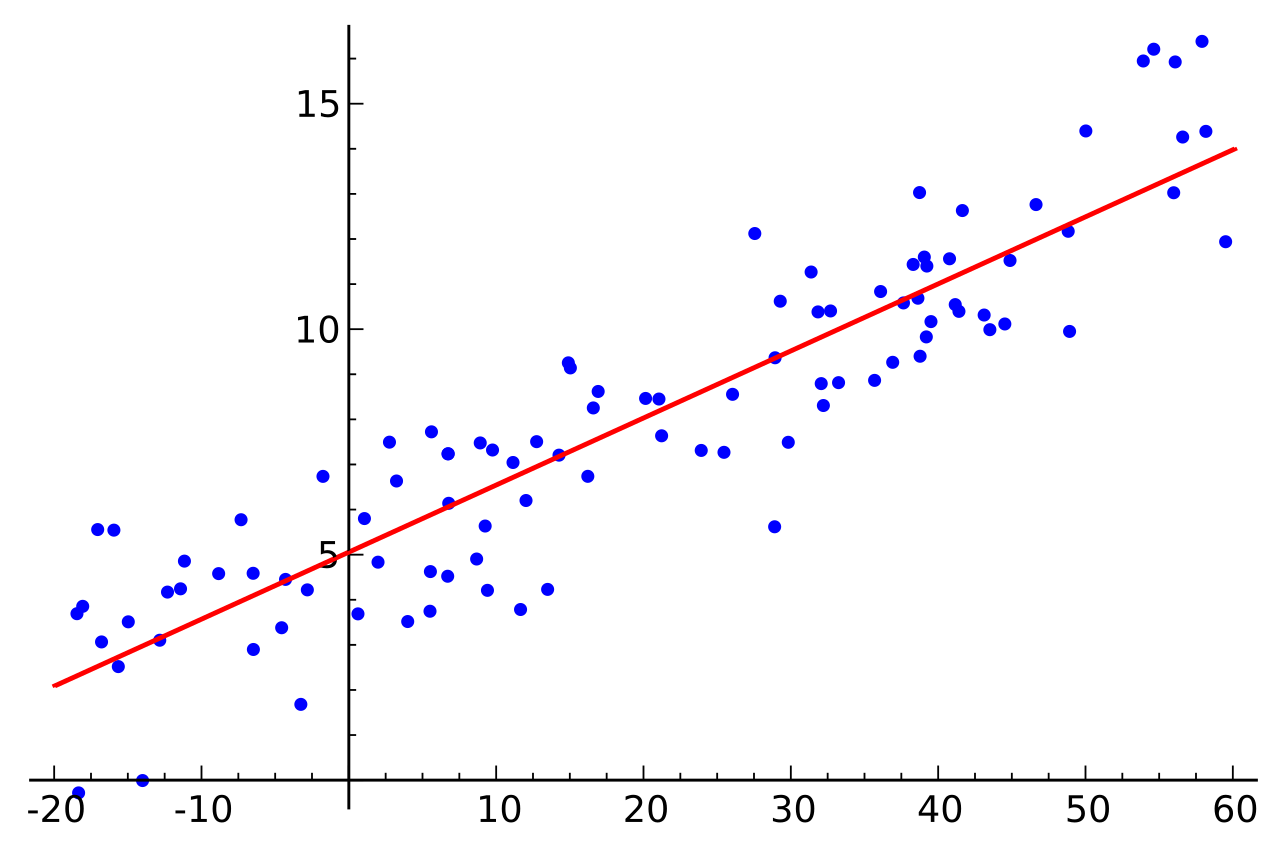
It also represents the variation between the observed and true realization of ‘y’. The expression ‘ε’ is the unobservable error that accounts for the inability of the data to stay on the straight line. The general term for these parameters is known as regression coefficients. The β 0 parameter is regarded as an intercept term, while the β 1 parameter is regarded as the slope parameter. The expressions β 0 and β 1 are the parameters of the linear regression model. In the simple linear regression model, y refers to the study or dependent variable and X is the explanatory or independent variable. Keep in mind that it becomes a multiple linear regression model when there are more than one independent variables. Usually, the model is typically called a simple linear regression model when there is just a single independent variable in the linear regression model. In the simple linear regression model, we consider the modelling between the one independent variable and the dependent variable. Basically, the simple linear regression model can be expressed in the same value as the simple regression formula.
